Sentiment Analysis: When Machines Can Beat Humans
Guest blogger Dr. Taras Zagibalov of Brandwatch addresses criticisms leveled at automatic sentiment analysis used for social media monitoring. Humans, he posits, often struggle to determine the sentiment of a piece of text because, just like the machine, they do not have the relevant knowledge available. Have current developments in machine analysis improved the process when compared with human analysis? And what of its own, inherent limitations? One perspective — after the jump.
Dr. Taras Zagibalov holds a doctorate in Informatics and heads the natural language processing research team at social media monitoring company Brandwatch. His work centers on improving real-time sentiment analysis to deliver accurate and up to date information for brands.
It’s not hard to find criticism of automatic sentiment analysis. Many of the most persuasive examples focus on illustrating how poor machines are at understanding emotions expressed through the complexities of human language. In some ways, they’re right.
Why?
Because these expressions are often only fully comprehensible with additional contextual and background information – information that may not be available to the machine.
As
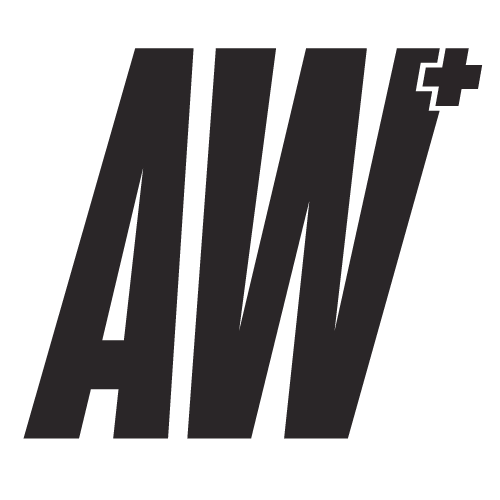
WORK SMARTER - LEARN, GROW AND BE INSPIRED.
Subscribe today!
To Read the Full Story Become an Adweek+ Subscriber
Already a member? Sign in