Pinterest Turns to Deep Learning to Generate Related Pins
When it comes to generating Related Pins, board co-occurrence is out and deep learning is in.
When it comes to generating Related Pins, board co-occurrence is out and deep learning is in.
Kevin Ma, a software engineer on Pinterest’s Discovery team, detailed the change in a blog post, explaining the logic behind the switch as follows:
One of the most popular ways people find ideas on Pinterest is through Related Pins, an item-to-item recommendations system that uses collaborative filtering. Previously, candidates were generated using board co-occurrence–signals from all the boards a Pin is saved to.
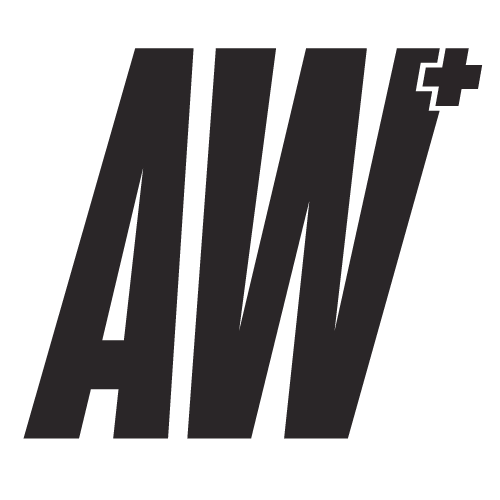
WORK SMARTER - LEARN, GROW AND BE INSPIRED.
Subscribe today!
To Read the Full Story Become an Adweek+ Subscriber
Already a member? Sign in