The experimental tool seemed innocuous enough at first glance: Plug in a pixelated image of a face and it would generate a higher-resolution mock-up using machine learning.
But it wasn’t long after an independent programmer posted it to Twitter last week that other researchers started to notice a glaring flaw. When prompted with Barack Obama’s blurry likeness, it returned a white man’s face with little resemblance to the former president. Ditto for other prominent people of color such as U.S.
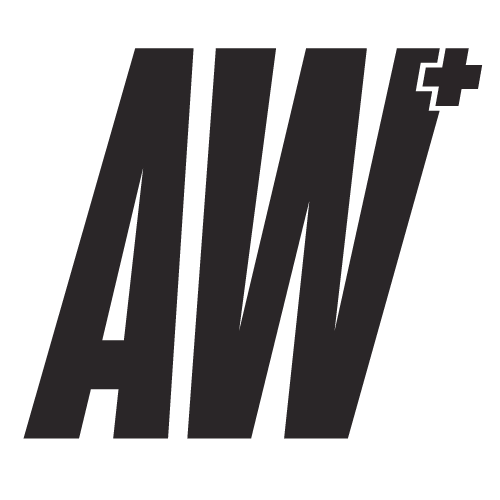
WORK SMARTER - LEARN, GROW AND BE INSPIRED.
Subscribe today!
To Read the Full Story Become an Adweek+ Subscriber
Already a member? Sign in