Despite a growing push in the artificial intelligence community to root out the human biases baked into many algorithms, a recent study from Microsoft researchers found that efforts to address these problems have often failed to account for the true nature and scope of structural racism.
The authors analyzed 146 recent papers published on bias built into natural language processing AI models and found that they often failed to sufficiently define bias or the relationship between language and entrenched societal hierarchies.
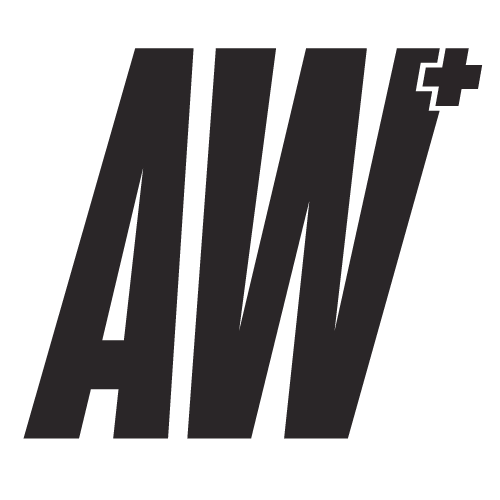
WORK SMARTER - LEARN, GROW AND BE INSPIRED.
Subscribe today!
To Read the Full Story Become an Adweek+ Subscriber
Already a member? Sign in