Inspiration meets innovation at Brandweek, the ultimate marketing experience. Join industry luminaries, rising talent and strategic experts in Phoenix, Arizona this September 23–26 to assess challenges, develop solutions and create new pathways for growth. Register early to save.
Research group OpenAI set the artificial intelligence community abuzz when it released a new paper on the latest version of its cutting-edge language generation system, GPT-3. The model was trained on a dataset more than 100 times larger than the already record-breaking amount of text that informed the previous version, GPT-2.
While OpenAI has yet to make the code behind GPT-3 publicly available as it did with GPT-2, the results of experiments outlined in the study show big improvements in the AI’s ability to generate realistic-sounding news articles and other text.
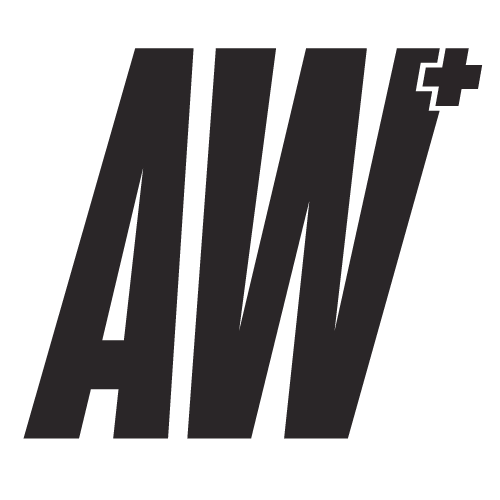
WORK SMARTER - LEARN, GROW AND BE INSPIRED.
Subscribe today!
To Read the Full Story Become an Adweek+ Subscriber
Already a member? Sign in