How Facebook Manages A 300-Petabyte Data Warehouse, 600 Terabytes Per Day
How did Facebook manipulate the Hive storage format to enable it to deal with a data warehouse that stores some 300 petabytes and takes in about 600 terabytes per day? RCFile (record-columnar file format) wasn’t enough, so enter ORCFile.
How did Facebook manipulate the Hive storage format to enable it to deal with a data warehouse that stores some 300 petabytes and takes in about 600 terabytes per day? RCFile (record-columnar file format) wasn’t enough, so enter ORCFile.
Pamela Vagata and Kevin Wilfong of the Facebook Analytics Infrastructure team described the creation of ORCFile in a post on the social network’s engineering blog.
Vagata and Wilfong described the motivation behind ORCFile:
There are many areas we are innovating in to improve storage efficiency for the warehouse — building cold storage data centers, adopting techniques like RAID in HDFS to reduce replication ratios (while maintaining high availability), and using compression for data reduction before it’s written to HDFS.
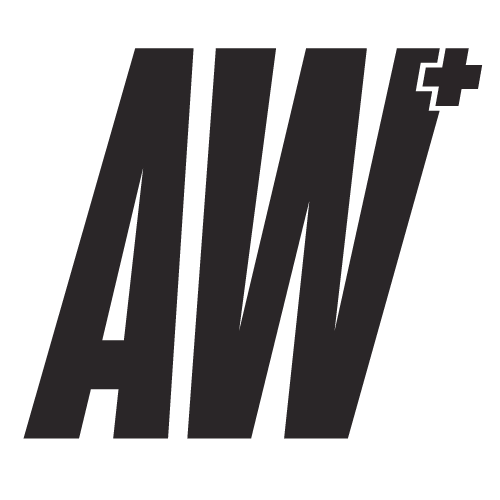
WORK SMARTER - LEARN, GROW AND BE INSPIRED.
Subscribe today!
To Read the Full Story Become an Adweek+ Subscriber
Already a member? Sign in