How To Not Screw Up A / B Testing Your App
This is a guest post by Tim Levine, the data guru at SocialMedia.com.
How many of your app design discussions have ended with: “Okay, let’s test it”? Hopefully you didn’t answer ‘none’, because testing is the fastest way to learn. But it can also misinform business decisions if done carelessly. Instead, I want to help you be careful in the simplest and most common kind of test: The A/B test. Of course, a well-designed multi-variate test is the best use of resources, but we have to roll over before we can crawl.
The
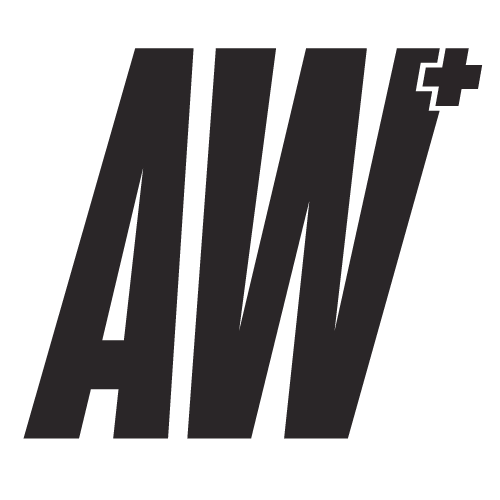
WORK SMARTER - LEARN, GROW AND BE INSPIRED.
Subscribe today!
To Read the Full Story Become an Adweek+ Subscriber
Already a member? Sign in